Machine learning approach led to the first iron climatology
Based on 32,344 dissolved iron data together with environmental predictors from contemporaneous satellite observations and reanalysis products, Huang and co-workers (2022, see reference below) propose the first data-driven surface-to-seafloor dissolved iron (Fe) climatology (1°×1°resolution). Their tools were 3 machine learning approaches among them the “random forest” method was tested as the most performant. Based on this climatology and statistical tools, the authors confirmed that atmospheric iron input plays a major role in enriching the surface tropical waters. They also show that interplay between particle remineralization, scavenging, and current transport are controlling the deep-water Fe distribution. Their analysis allows them to objectively assess the caveats and limitations of this approach. However, they eventually propose to use this climatology to evaluate the performance of the ocean biogeochemical models (OBM). With this aim, 13 process-based models are tested against data-driven climatology.
Global Dissolved Fe Dataset and Model Performance
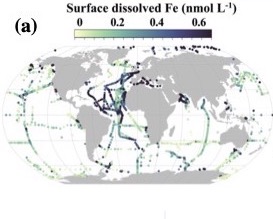
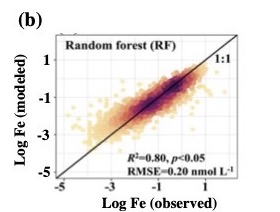
Global Projection
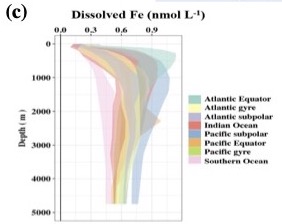
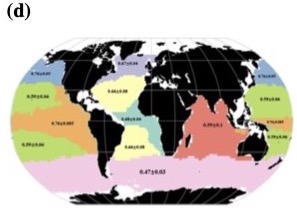
Evaluation of process based Model
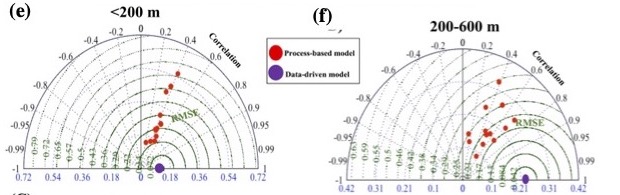
Figure: a) Distribution of compiled global observation dataset of dissolved Fe with the respect to surface ocean. b) Model performance evaluated with the validation dataset. c) Mean profile of dissolved Fe (dFe) with the shading representing the standard deviation. d) Mean dFe concentration at the depth between 3000 m and 3500 m in the main ocean basin. Diagrams comparing global modeled dissolved Fe from thirteen existing process-based models against the random forest data-driven model at the depth different depth intervals e) above 200 m and f) between 200 m and 600 m.
Reference:
Huang, Y., Tagliabue, A., & Cassar, N. (2022). Data-Driven Modeling of Dissolved Iron in the Global Ocean. Frontiers in Marine Science, 9. Access the paper:10.3389/fmars.2022.837183